Ecommerce recommendations are a significant part of cross-selling, upselling, and sustaining customer engagement. Recommendations are relevant to anything that users and buyers consume, from products and solutions, to entertainment, and even news. One way to make recommendations to your customers would be to recommend items that are similar to items already browsed or purchased by the customer.Â
However, merchants might want to showcase a larger cross-section of products to their customers, or might want to also draw the customer to view other product categories. Additionally, in some cases, it might not be foolproof: a customer who just purchased a collection of succulent plants or accounting software might not want to keep getting recommendations for succulents and additional accounting software. They already have this. What next?Â
Enter AI-powered recommender systems, an intelligent way of making ecommerce recommendations. But before we dive into the nitty gritty of AI recommendations, do you really need to care about product recommendations at all? And if yes, why?Â
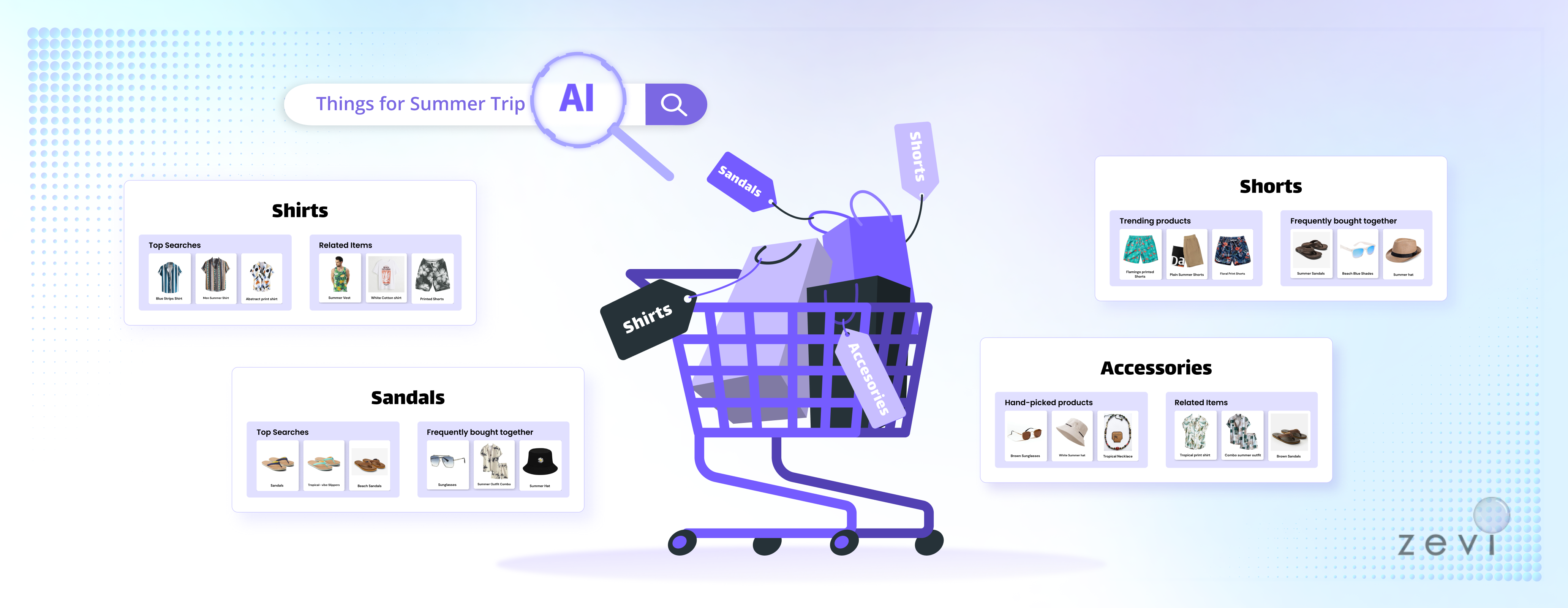
Product recommendations – the key to memorable online shopping experiencesÂ
Recommendations are crucial to a positive user experience, and also to give merchants a platform to showcase the scope of their product offering. Over one-third of Amazon’s sales come from product recommendations. Anyone who has shopped on Amazon has received “other items you might like”, “other users also purchased” or “frequently bought together” recommendations. Sephora makes recommendations based on what is trending. Netflix tells users what will be an X% match based on what users who viewed similar series and movies.Â
Customers visit a website either knowing full well what they want to purchase, like a “remote-controlled car for a 5-year-old boy” or “Cat food for a 6-month-old kitten”. However, they might also be looking for a gift, or might just be browsing for decorative items (or must-haves) for the new home. Recommendations can go a long way in making your customer experience smoother for the browsers, but they can also help those who come to your website know what they want.
For example, you might recommend a litter tray to the cat food seeker and a coloring book or lego blocks for the shopper looking for items for a 5-year-old.Â
An Ecommerce Personalization Benchmark Report 2021 conducted by researcher Wakefield, and Netcore, discovered that: 91% of customers would abandon an online retailer over a poor shopping experience. More than 75% said that merchants needed to personalize the experience to fit their preferences. And yet, less than half (41%) of retail executives say their ecommerce platform is only “somewhat personalized”. Only 13% of online retailers boasted a completely personalized experience. Of course, one can assume that these figures have improved somewhat in the last year or so, but clearly, there is a gap in the market. Moreover, there is a clear opportunity. Over 70% of retailers who invested in personalizing customer experience witnessed an increase in conversion rate that exceeded 40%.
Using AI to build product recommendationsÂ
Today ecommerce merchants have machine learning and advanced AI algorithms to fine-tune product suggestions. Of course, not every online retailer has Netflix or Amazon’s deep pockets and other resources, and therefore you might not have access to the extremely intelligent (and well-trained!) AI algorithms it uses.Â
Collaborative filtering can be a cost-effective way to get personalized recommendations, and thereby improve customer satisfaction and retention, while also identifying opportunities to cross-sell and upsell.Â
So what exactly is collaborative filtering? We’ll get to the technical definition, but let’s look at the popular layman-friendly Wikipedia definition to ease in. Wikipedia defines collaborative filtering like this:Â
‍Collaborative filtering is a method of making automatic predictions (filtering) about the interests of a user by collecting preferences or taste information from many users (collaborating). The underlying assumption of the collaborative filtering approach is that if person A has the same opinion as person B on an issue, A is more likely to have B's opinion on a different issue than that of a randomly chosen person.Â
As you can see, if buyer A liked or purchased products 3, 5, and 8; collaborative filtering will assume that buyer B, who also liked or purchased products 3 and 5, might also like – and buy – product 8.Â
The more technical definition of collaborative filtering goes like this:Â
‍Collaborative filtering identifies customer similarities based on site-based behavior and then uses this information to personalize product recommendations.Â
Types of collaborative filtering
‍User-basedÂ
Here, recommendations are driven by ratings on behavior of other users who have displayed similar behavior to the user whom you are recommending to.Â
For example, Jim picks several white shirts and a dinner jacket. Other users who pick these items often pick bow ties and cufflinks. The site will recommend bow ties and cufflinks to Jim.Â
‍Item-based
Amazon pioneered this type of filtering back in 1998, and you guessed it, it's still in use, even if the e-commerce giant now has better technology driving its filtering. Here, recommendations are driven by the user’s buying behavior and interests. If Linda tends to click on and purchase knee-length skirts, her recommendations will show her more knee-length skirts.
‍Matrix factorizationÂ
In this type of collaborative filtering, the mathematical concept of matrix factorization is used to make recommendations. An entity is categorized into smaller entities, using a table. From this, one can draw correlations or discover how entities interact with one another. It would look something like this, and in cases where a value is not inputted or known (because the user hasn’t provided a rating), the machine learning algorithm assumes the value to be zero.Â
‍HybridÂ
Of course, depending on what your product or solution is, you might want to use a combination of one or more of the above types of collaborative filtering. Work with your solutions provider to evaluate what type of collaborative factoring will work best for your unique business needs.Â
Wrapping up
Personalized recommendations are no longer an option if 9 out of 10 customers are willing to walk away from retailers on account of the lack of it. Fortunately, ecommerce merchants have an effective way to deliver personalizations, by various types of collaborative factoring, that help make recommendations about the user’s behavior and behavior of other users, or by tallying up the two.Â
With Zevi, you can improve site search by harnessing the power of NLP. This means that you can enjoy Netflix/Amazon standards at a fraction of the cost. NLP recognizes Hinglish (Hindi with English) and Spanish with English search, cues, and results among other foreign languages.
Watch Zevi in action – sign up for a free demo today!Â