In the past, search engines would match search queries based on specific keywords or phrases. However, nowadays, the focus has shifted to understanding what your customers are looking for.Â
An example is a user searching for "coffee shops near me." In the past, search engines would return results based on the user's location and the keyword "coffee shops."Â
However, the results were not guaranteed to match the user's intent. The user could be looking for a quiet place to work or a cozy atmosphere to hang out with friends. Nowadays, search engines can use AI and NLP to identify the user's intent based on their search history, browsing behavior, and location data.Â
For instance, if a user frequently searches for cafes with free Wi-Fi, the search engine can infer that the user is looking for a quiet place to work. Based on the user's intent, search engines can provide more relevant and accurate results that match their search query.
This blog will discuss how AI and ML models can help you accurately identify your customers' search intent and offer them a seamless online experience.
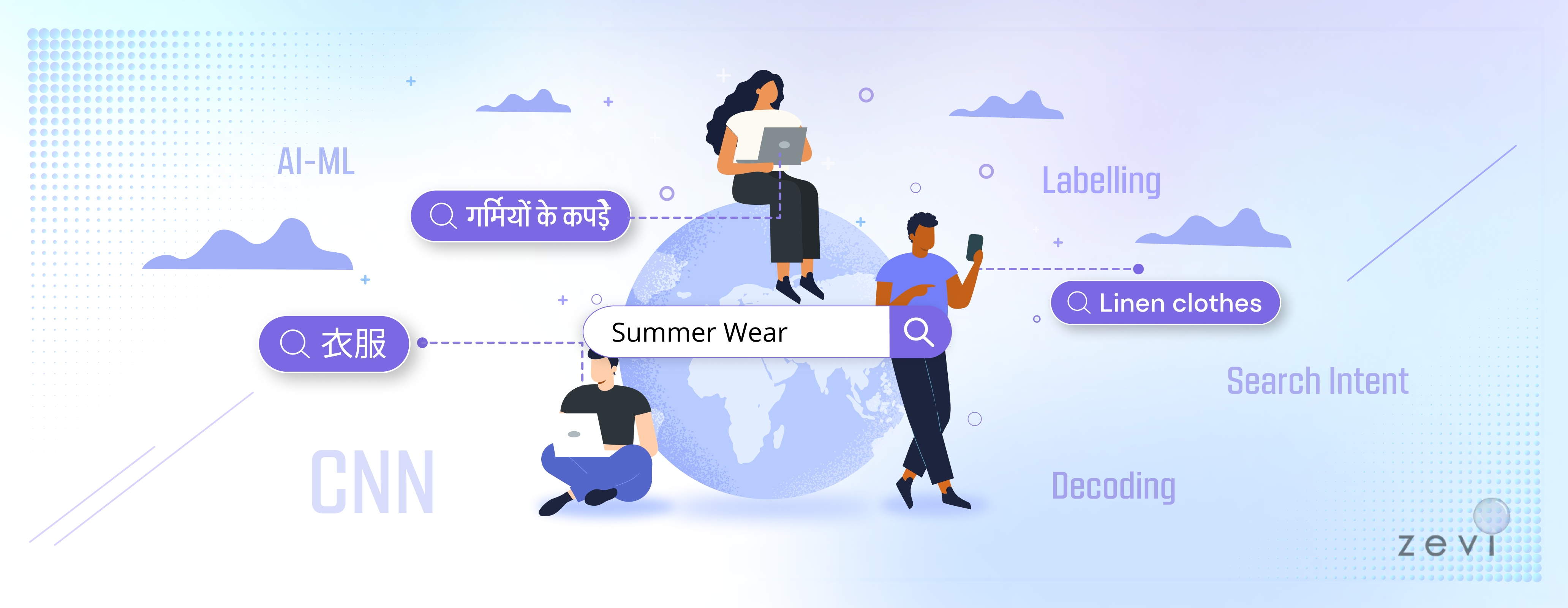
User intent - The key to effective searchÂ
Search engines have evolved over the decades, with the focus shifting from keyword or string matching to understanding the user's intent. Why?
In the early days of search engines, users would enter keywords or phrases into a search box. The engine would return a list of pages containing those exact words or phrases. However, this method didn't always provide the most relevant results because the search engine couldn't understand the context or intent behind the query.
Enter natural language processing (NLP) – NLP is a subfield of artificial intelligence that focuses on the interaction between computers and human language. It allows machines to understand, interpret, and generate human language.Â
As search technology advanced, search engines began using more sophisticated algorithms that could analyze the context and intent behind a user's search. This allowed them to deliver more accurate and relevant search results, which improved the user experience.Â
Today, search engines use artificial intelligence and machine learning to analyze vast amounts of data, including the user's search history and browsing behavior, to better understand their intent and provide personalized results. This evolution has been driven by the need to provide users with the most relevant and valuable information possible and to stay competitive in a crowded marketplace.
Intent can be classified into four types:Â
- Informational intent: This type of intent is all about seeking information. Users with an informational intent want to learn more about a particular topic or answer a specific question. For example, a user might search for "how to make homemade pizza" or "what are the symptoms of the flu."
- Navigational intent: Navigational intent is when a user searches for a specific website or page. For example, a user might search for "Facebook login" or "Amazon Prime Video."
- Transactional intent: This type of intent is focused on buying or transacting. When a user has transactional intent, they want to make a purchase or complete a specific action. For example, a user might search for "buy shoes online" or "subscribe to Netflix."
- Consideration intent: Consideration intent is when a user explores their options before making a purchase. When a user displays consideration intent, they are researching different products or services to determine which one is the best fit for their needs. For example, a user might search for "best smartphones under $500" or "top-rated laptops for college students."
Why businesses need to focus on user intent
By focusing on user intent, you can ensure that your digital marketing efforts are directed towards the right audience and the right stage in their buying journey. This means that you can tailor their messaging and content to meet the specific needs of their audience, resulting in a more personalized and engaging experience for users.
Plus, with the rise of voice search and virtual assistants, user intent has become even more critical. As users increasingly use voice search to make queries, you need to ensure that your content not only ranks well in search engines but also provides a seamless experience for voice search users.
Picture this – a potential customer is searching for a dress for a special event. They got to your site and searched for "party dress cattleya". Now you might not have any dresses tagged with 'cattleya'. If you don't offer any alternative suggestions or recommendations for similar dresses – it's game over. The customer will leave your site.
Now, imagine if your search engine could understand the user's intent, and offer alternative suggestions based on the search query. You could have recommended similar dresses in shades of purple or offered accessories that would complement the dress the customer initially searched for. This would not only provide a better user experience but also increase the likelihood of a successful transaction.
Decoding intent with Artificial Intelligence and Machine Learning models
Humans define intent based on our understanding of the language and the context of the conversation. We use our knowledge, experience, and common sense to interpret the meaning of the words and phrases used in the conversation.
On the other hand, AI and ML models rely on statistical patterns and mathematical models to make sense of language and the meaning behind words.Â
Let's dive into the technical details of how that happens:Â
Vectors are mathematical representations of words and phrases that capture their meaning. Each word or phrase is assigned a vector representing its semantic meaning. These vectors can be used to calculate the similarity between different queries and documents.
For instance, if a user searches for "best smartphones," the AI model converts the query into a vector and searches for documents with similar vectors. The documents with similar vectors are considered relevant to the user's search query and returned as search results.
There are two methods to train the AI: manual labeling and auto-labeling.Â
Manual labeling involves a team of humans manually labeling data sets, which are then fed into the AI for training. Auto-labeling involves using unsupervised learning techniques like clustering, where similar queries are grouped together, and the labels are automatically generated.
To effectively analyze textual data and extract meaningful insights, it's crucial to use advanced neural network architectures. One such architecture is the Convolutional Neural Network (CNN),
CNNs are a powerful type of neural network used for intent analysis. They have proven to be highly effective in identifying patterns in textual data, making them a valuable tool in various NLP applications, including intent analysis.
The magic of CNNs lies in their ability to extract relevant features from the input text and learn representations that help identify intent. For example, if a user searches for "coffee shops near me," the CNN model would extract features such as location, distance, and reviews from the input text. Using these learned representations, the model would identify the user's intent and return relevant search results that match their query.
In other words, CNNs use a layered approach to analyze text and identify patterns. The first layer detects basic features such as lines, edges, and corners. As the layers progress, the network learns more complex characteristics such as shapes, objects, and concepts. Finally, the last layer identifies the intent behind the user's query.
Conclusion
Overall, AI and ML models have revolutionized search functionality, making it possible for businesses to accurately decode user intent and provide personalized experiences. By leveraging these technologies, you can better understand your customers' needs and preferences, improve customer engagement, and ultimately drive more conversions.
If you're looking for the best-in-class AI-based search engine – we've got you covered.
With Zevi, businesses can build intelligent search experiences that enhance engagement, increase customer satisfaction, and drive revenue growth. Whether you're an e-commerce retailer, a media platform, or a SaaS company, Zevi can help you unlock the power of AI to deliver unparalleled search experiences to your users. Opt for a free demo today!